The Competitive Chasm: Act Now or Fall Behind?
The global AI market is at a turning point, a moment where decisions today will shape outcomes for years to come. Imagine this: in 2024, the market is valued at $279 billion, but by 2030, it’s projected to soar to $1.8 trillion, growing at a stunning annual rate of 35.9% (Grand View Research, 2024). This isn’t just a a story about growth. This is a fundamental shift in how businesses compete.
Think about it: 72% of companies now use AI in at least one business function, and 65% report regularly using generative AI (McKinsey Global Survey on AI, 2024). Yet, only 26% have scaled beyond pilot programs to achieve real business value (Boston Consulting Group AI Transformation Study, 2024). This gap between adoption and implementation creates a critical fork in the road. For some, it’s an opportunity for long-term leadership; for others, it’s an oncoming risk of being left behind.
The Executive Blind Spot: Are We Seeing the Whole Picture?
Here’s a scenario that might sound familiar: C-suite executives think only 4% of employees use generative AI for a significant portion of their daily work. But when employees were asked directly in the same McKinsey survey, they reported much higher usage rates. Sound like a miscommunication? It’s more than that; it’s a blind spot that could derail strategy and leave organizations exposed.
Why does this matter? Employees are already using AI tools, often without formal guidelines or governance. It’s like trying to drive a race car without a track or rules, it’s risky, chaotic, and can lead to unintended (devastating ) consequences. Deloitte’s 2024 report on generative AI highlighted the operational, legal, and reputational risks of this shadow AI adoption. And yet, many C-suites haven’t fully grasped the scale of the issue.
CEO Involvement: The Game-Changer
When was the last time you saw a CEO roll up their sleeves and get involved in AI strategy? According to McKinsey’s 2024 Global AI Survey, companies with CEOs directly overseeing AI not only move faster but also see stronger financial results, impacting earnings before interest and taxes (EBIT) in a measurable way.
Yet, only 28% of companies report this kind of hands-on leadership. This gap is telling. Without strategic alignment at the top, organizations stumble into common pitfalls:
- AI programs that don’t align with business goals
- Wasted resources on duplicated efforts
- Higher risks from uncoordinated AI use
- Slower time-to-value without executive support
The takeaway? Leadership had always been about more than just approving budgets. Captains propser when they are actively steering the ship. Executives need to get caught up on the key elements that make up AI Governance, and have their organizations cultural adoption start at the top.
The Numbers Don’t Lie: The Revenue and Risk Equation
The case for AI is both exciting and clear. Across industries, AI is transforming how companies grow and operate. Let’s break it down:
Revenue Growth:
- 70% of financial institutions report AI-driven revenue increases of over 5% (NVIDIA AI in Financial Services Survey, 2025).
- Retailers using AI see average revenue jumps of 19% (IBM Global AI Adoption Index, 2024).
- AI-powered product recommendation engines boost revenue by 15-30% (Salesforce State of Commerce Report, 2024).
- Advanced personalization increases average order values by 40% (Adobe Digital Economy Index, 2024).
Operational Efficiency:
- AI can cut costs by up to 40% across industries (McKinsey Global Institute, 2024).
- Workflow automation reduces repetitive tasks by as much as 75% (Deloitte Future of Work Survey, 2024).
- AI-powered customer service slashes costs by 47% (Zendesk Customer Experience Trends Report, 2024).
- Optimized supply chains reduce operational costs by 15% (Gartner Supply Chain Technology Survey, 2024).
These aren’t just statistics; they’re proof that AI, when used strategically, can unlock new levels of performance and efficiency.
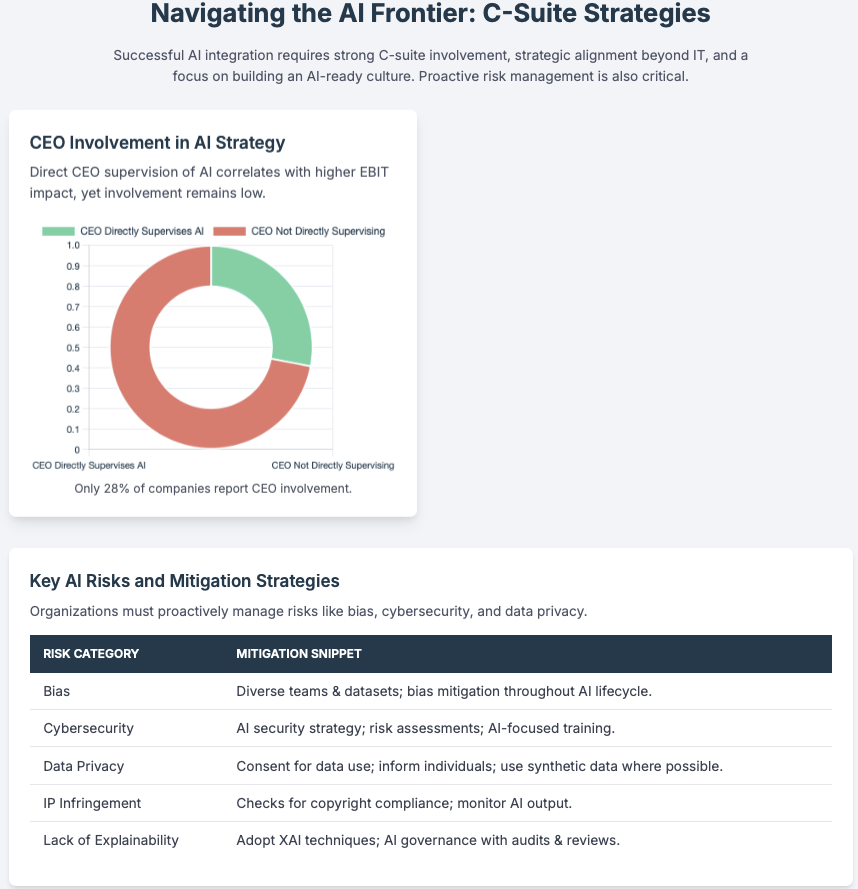
Facing the Risks: Why Governance Matters
It’s tempting to focus on the rewards AI can bring, but the risks are just as real. Without proper governance, organizations leave themselves open to regulatory, operational, and reputational liabilities. You wouldn’t want to build a house without a blueprint would you? It might stand for a while, but it’s bound to crumble without a solid foundation.
As we navigate this AI-driven future, the question isn’t just “How can we adopt AI?” but “How can we do it responsibly and effectively?” The answers lie in leadership, strategy, and a willingness to address the challenges head-on. For assistance in these areas, Tech Jack Solutions offers advisory services here: AI Governance Service Overview
Understanding these risks requires a systematic approach. Leading organizations are adopting structured frameworks that map specific exposures to regulatory requirements:
AI Risk Management Framework (Based on NIST AI RMF 1.0)
Risk Category | Specific Exposures | Regulatory Alignment |
Govern | Lack of AI oversight, unclear accountability structures | NIST AI RMF: Governance function |
Map | Unknown AI systems in use, shadow AI adoption | EU AI Act Article 9: Risk management requirements |
Measure | Bias in AI outputs, fairness violations | NIST SP 1270: Socio-technical approach to bias |
Manage | Data privacy breaches, IP leakage, safety incidents | CSA Core Security Responsibilities |
Bias and Ethics Considerations: The NIST Special Publication 1270 establishes that AI bias emerges from socio-technical systems, not merely technical artifacts. This framework recognizes that bias mitigation requires addressing both algorithmic design and organizational processes. CSA’s Core Security Responsibilities paper further emphasizes that bias detection and mitigation must be embedded throughout the AI lifecycle, from data collection through model deployment and monitoring.
Sector-Specific Imperatives isk management sophistication.
How This Plays Out in Regulated Sectors
When it comes to AI governance, context is everything. Different sectors face unique challenges, and it’s worth reflecting on a few examples to unpack this.
Healthcare: Consider the intersection of HIPAA compliance and AI governance. Here, the FDA’s Software as a Medical Device framework isn’t just a guideline, it’s a roadmap to ensure that AI models are validated and bias is tested. It’s a balancing act between innovation and patient safety.
Financial Services: In this sector, managing risk is second nature. The OCC’s guidance on model risk management extends naturally to AI systems, while Federal Reserve supervisory expectations highlight explainability. Imagine the importance of clear, logical AI-driven decisions, especially when it comes to something as personal as credit approvals.
Critical Infrastructure: Here, the stakes are even higher. CISA’s AI roadmap emphasizes securing the supply chain for AI components and third-party services. It’s not just about protecting data, it’s about safeguarding the systems that keep our society running.
The Imperative: Understanding the Cost of Competitive Inaction
AI Governance: A Strategic Enabler
Let’s address a common misconception: AI governance is often seen as a roadblock to innovation. But what if we flipped that narrative? A well-thought-out governance framework can actually accelerate progress, acting as a guide rather than a gatekeeper.
Building for Rapid, Responsible Innovation
Think of it like setting up the rules for a game, clear boundaries allow for creativity and experimentation within a safe space. This means:
- Defining parameters for testing ideas without crossing into risky territory
- Streamlining processes to evaluate, scale, and improve AI initiatives
- Building ethical practices into the DNA of every project
Reducing Risk Across the Organization
Governance is about rules and protection. A system that proactively detects bias (aligned with NIST SP 1270), keeps up with evolving regulations like the EU AI Act, and ensures that accountability is crystal clear. Add in robust audit capabilities, and you’ve got a roadmap for not just compliance but trust.
The competitive landscape is experiencing rapid structural shifts. Organizations that establish AI leadership today, through strategic adoption combined with comprehensive governance, will capture disproportionate market advantages in efficiency, innovation capability, and customer experience delivery.
Key Performance Indicators: Tracking Progress
How do you measure success in AI leadership? Positive AI outcomes are great, but there is also value that is realized within journey itself. Measuring AI leadership requires both forward-looking indicators that predict success and outcome metrics that demonstrate results. Based on the GAO AI Accountability Framework, executives should track:
Leading Indicators (Predictive)
These metrics help you spot trends and address issues before they escalate:
- What percentage of AI models are fully documented with model cards? (Target: 100%)
- How fast can you detect bias or AI incidents? (Target: less than 24 hours)
- Are AI projects passing fairness tests before deployment? (Target: 100%)
- How many employees are equipped with AI literacy? (Target: 80% within a year)
Lagging Indicators (Outcomes)
These reveal the real-world impact of your AI efforts:
- How much of your revenue comes from AI-driven initiatives?
- What cost savings can be attributed to automation?
- Are there any compliance violations? (Target: zero)
- Are customers satisfied with AI-enhanced services?
Companies that delay decisive action will increasingly find themselves competitively disadvantaged across multiple dimensions: operational efficiency, decision-making speed, customer personalization capabilities, and risk management.
Strategic Action Framework: Five Steps for C-Suite Leaders
Adopting AI comes with its challenges and opportunities. Based on lessons from successful implementations, there’s a clear way to approach it with a mix of innovation and strategy. Here are five actionable steps for leadership teams:
- Establish Executive AI Governance
Every transformation starts with leadership. Think of this as building the guardrails before you hit the accelerator. By forming an AI strategy council with clear authority and regular updates to the board, you create alignment at the top, a foundation that ensures every decision and action stays on course.
- Conduct an Organizational Assessment
Before rushing ahead, take a moment to reflect: Where are we today? This step is like checking the map before embarking on a road trip. Using tools like the NIST AI RMF, evaluate your current AI usage, data readiness, and gaps in your capabilities. Knowing your starting point helps you chart a smarter path forward.
- Build a Governance Framework
This is about creating a safety net for innovation. Imagine a tightrope walker. Without a net, every step feels riskier than it needs to be. A governance framework, with ethics committees, risk management protocols, and compliance monitoring, ensures you can take bold steps confidently and responsibly.
- Invest in Capability Building
At its core, AI is about empowering people. Think about it like assembling a team of mountain climbers before scaling a 14k’er. Roll out literacy programs, identify high-impact use cases, and align them with your broader strategic priorities. The more prepared your team is, the higher you can climb together.
- Launch Strategic Pilot Programs
Start small, but keep the bigger picture in mind. Pilot programs are like dipping your toes in the water before diving in. By setting clear goals, measurable outcomes, and ensuring scalability, these small steps pave the way for bigger transformations.
The challenge for C-suite leaders isn’t just about whether to adopt AI. That decision’s already been made by the pace of the market. Instead, the real question is: How quickly and thoughtfully can we build these capabilities? The opportunities are vast, but the window to gain a competitive edge is narrowing fast.
AI governance should be thought of as a checkbox exercise. It’s a tool for creating trust, unlocking value, and fostering innovation. When done thoughtfully, it safeguards what matters most while empowering the people and systems it serves. After all, AI is only as good as the foundation it’s built on.
In the next part of this analysis, we’ll explore specific governance frameworks, implementation strategies, and performance measurement tools that can help organizations adopt AI responsibly and at scale, minimizing risks while maximizing business value.
Primary Sources and References:
- Grand View Research. “Artificial Intelligence Market Size, Share | Industry Report, 2030.” 2024.
- McKinsey Global Institute. “The Economic Potential of Generative AI: The Next Productivity Frontier.” 2024.
- McKinsey & Company. “Global Survey on the Current State of AI.” 2024.
- NIST. “AI Risk Management Framework (AI RMF 1.0).” NIST AI 100-1. 2023.
- NIST. “Towards a Standard for Identifying and Managing Bias in Artificial Intelligence.” NIST SP 1270. 2022.
- Deloitte. “State of Generative AI in the Enterprise.” Q4 2024.
- NVIDIA. “AI in Financial Services Survey.” 2025.
- Cloud Security Alliance. “AI Safety Initiative: Core Security Responsibilities.” 2024.
- Government Accountability Office. “Artificial Intelligence: An Accountability Framework for Federal Agencies and Other Entities.” GAO-21-519SP. 2021.
- EU AI Act. “Regulation on Artificial Intelligence.” Article 9: Risk management system. 2024.
This article is part of a comprehensive series on AI adoption and governance for C-suite executives. The next installment will provide detailed frameworks for implementing effective AI governance structures that accelerate value realization while managing enterprise risk.
Tech Jacks Solutions
May 25, 2025TJS