Artificial Intelligence (AI) is transformative, powerful, and potentially unsettling if left unchecked. If your organization’s approach to AI governance currently resembles an unstructured free-for-all, it’s critical to implement structured guidelines immediately.
Establishing a comprehensive AI Acceptable Use Policy (AUP) is essential not only to manage risks but also to demonstrate ethical responsibility and compliance with evolving regulations. Here’s an expanded guide to creating an effective AI AUP.
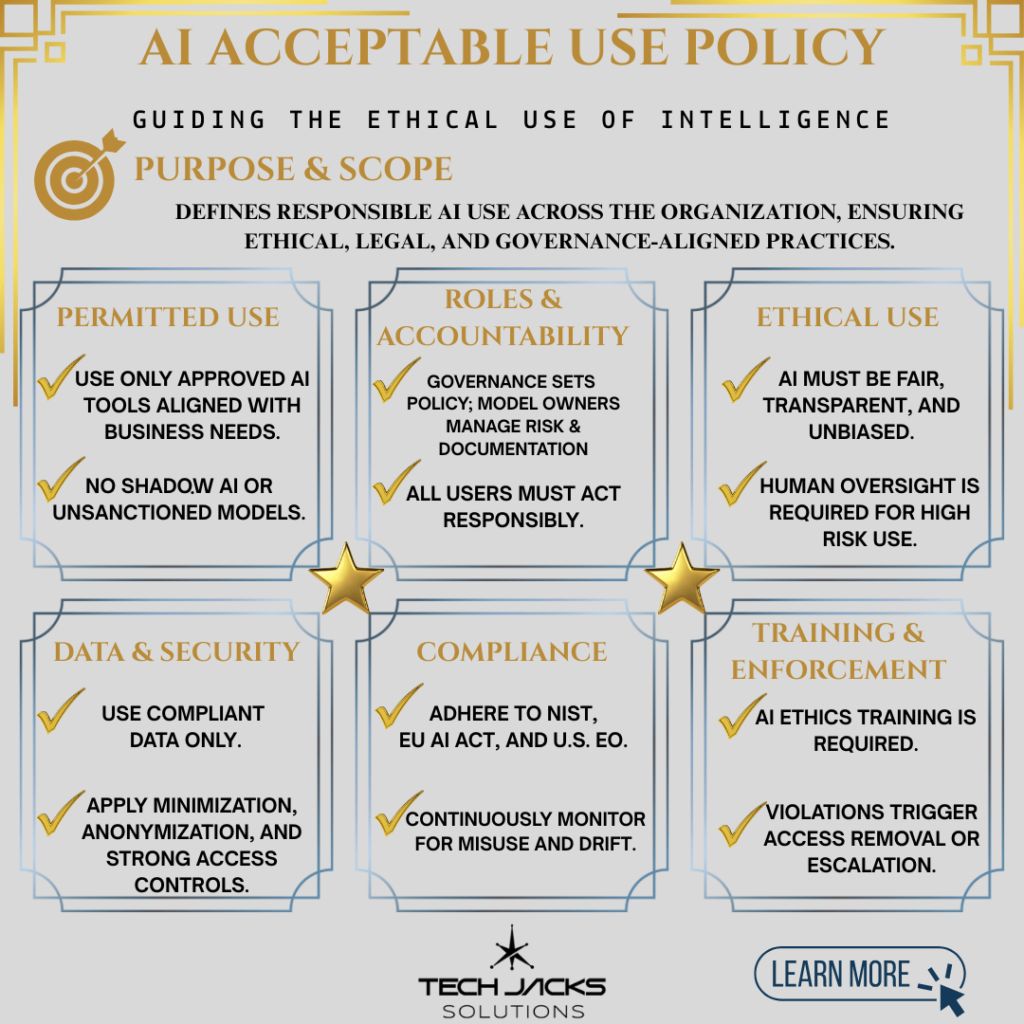
The Crucial Importance of an AI Acceptable Use Policy
As AI increasingly integrates into various business processes, the pressures from regulatory bodies, such as the EU AI Act, NIST AI Risk Management Framework, and state-specific AI laws, become more pronounced. Organizations need a well-structured AI AUP to navigate these complexities successfully, fostering both regulatory compliance and stakeholder trust.
Due diligence & Due care are real things. Without an AI Acceptable Use Policy, organizations run the risk of not performing Due care, which can exacerbate any penalty or non-compliance violation that may, and often will, occur.
Detailed Components of an Effective AI AUP
Purpose and Scope
Clearly articulate why the policy exists. Your AUP must precisely identify the goals, including compliance, ethical oversight, and risk mitigation. Outline explicitly which systems, data types, and users the policy covers, and clearly specify exclusions to avoid confusion.
The policy should define the high-level goals the organization wants to underline and promote regarding AI Usage.
Don’t forget, use additional policies your organization has already implemented and deployed to enhance and make your AUP more resilience. Leverage work already performed, referencing more authoritative policies and guidance as needed.
Definitions of Key Terms
Clearly define critical terms like “Generative AI,” “Sensitive Data,” “Algorithmic Bias,” and “High-Risk AI Use Cases.” Providing precise definitions helps maintain consistency and clarity across the organization.
Examples:
- AI Acceptable Use Policy (AUP): A document outlining the allowed and restricted ways in which artificial intelligence technologies can be used within an organization.
- Generative AI (GenAI): A type of artificial intelligence that can generate new content, such as text, images, audio, and video, based on the data it has been trained on.
- Ethical Principles (in AI): Fundamental values and guidelines that should govern the development and use of AI technologies, such as fairness, transparency, accountability, and beneficence.
- Data Privacy: The right of individuals to control how their personal information is collected, used, and disclosed. Regulations like GDPR and CCPA/CPRA aim to protect data privacy.
- Intellectual Property Rights: Legal rights that protect creations of the mind, such as inventions, literary and artistic works, designs, and symbols. Examples include patents, copyrights, and trademarks.
- Bias (in AI): Systemic errors in AI algorithms that result in unfair or discriminatory outcomes for certain groups of people. Bias can be present in training data or introduced during model development.
- Guardrails (in AI): Mechanisms and controls implemented to ensure that AI systems operate safely, ethically, and within acceptable boundaries. This includes filtering inputs and outputs.
- Compliance: Adherence to laws, regulations, standards, contractual obligations, and internal policies.
- Risk Management Framework: A structured approach to identifying, assessing, and controlling potential risks within an organization.
- Transparency (in AI): The extent to which the workings and decision-making processes of an AI system are understandable and explainable to humans.
Governance and Accountability Structures
Here is where most organization often have Kryptonite crumbled over their superhuman efforts. Lack of accountability/responsibility, or at the very least, not very clear RACI structures. Clearly outline roles and responsibilities to avoid ambiguity:
- Executive Sponsor: Responsible for overall AI strategy, policy enforcement, and allocation of resources.
- AI Governance Committee: Oversees AI use cases, risk assessments, and ethical reviews.
- Model Owners (Data Scientists/ML Engineers): Responsible for AI model development, validation, ongoing monitoring, and reporting.
- Data Steward: Ensures data privacy, quality, and compliance with data management standards.
- Legal & Compliance Teams: Advises on regulatory compliance, legal risks, and updates the policy according to legal changes.
Regular meetings and clear documentation ensure accountability and transparency.
Your accountability structure will also correspond to how model security and standards are implemented. When utilizing and developing models, security needs to be built in by design. Do not repeat mistakes of the past by taking the more expensive route of cramming security in afterwards. Perform & implement activities such as:
- Detailed Security Standards: Implement rigorous vulnerability assessments, adversarial testing frameworks, and runtime protections.
- Security Testing Protocols: Establish regular penetration testing and stress testing of AI models.
- Secure Runtime Environment: Mandate runtime monitoring and protections such as sandboxing or containerization to prevent unauthorized access or misuse.
Guidelines for Responsible AI Acceptable Use
Implement ethical guidelines based on principles such as fairness, transparency, privacy, and accountability. Require human oversight for critical AI-driven decisions like recruitment, performance reviews, financial credit evaluations, and healthcare diagnostics. Clearly distinguish between acceptable and unacceptable AI applications.
Activities that can be performed to help with this would be:
- Bias Pre‑screening: run training data through the NIST bias taxonomy checklist and document mitigations in each model’s “Data Sheet.
- Adversarial & Red‑Team Testing at three gates (pre‑prod, launch, quarterly) using the NIST AI 100‑2e attack taxonomy.
- Human‑in‑the‑Loop (“HITL”) Sign‑off for any AI output that drives legal, credit, safety, medical or employment decisions.
- Source‑Control Tagging & License Check for all training corpora (open‑source, public‑web, customer‑owned). Deny ingestion if usage rights are ambiguous.
- Sensitive‑Data Safeguards: automatically classify and redact PII/PHI before it reaches foundation‑model prompts; use differential‑privacy or synthetic data where feasible.
- Model Cards + Risk Cards posted on the internal wiki for every production model, covering purpose, limitations, datasets, known hazards and contact owner.
- User‑facing Explanation Mode for all customer‑visible AI decisions (e.g., adverse‑action notices, credit scores) that surfaces the top variables and confidence level.
Data Handling and Privacy Considerations
Establish rigorous data governance procedures:
- Restrict unauthorized data uploads or downloads.
- Enforce robust anonymization and pseudonymization practices.
- Maintain comprehensive access control protocols, encryption standards, and logging mechanisms to ensure data integrity and confidentiality.
- Source‑Control Tagging & License Check for all training corpora (open‑source, public‑web, customer‑owned). Deny ingestion if usage rights are ambiguous.
Risk Management and Ethical Review
Establish thorough ethical review processes for AI implementations, particularly high-risk cases. Develop and maintain a Risk Classification Matrix to prioritize AI reviews. Clearly define procedures for incident identification, escalation, and response to ensure swift action in crisis scenarios.
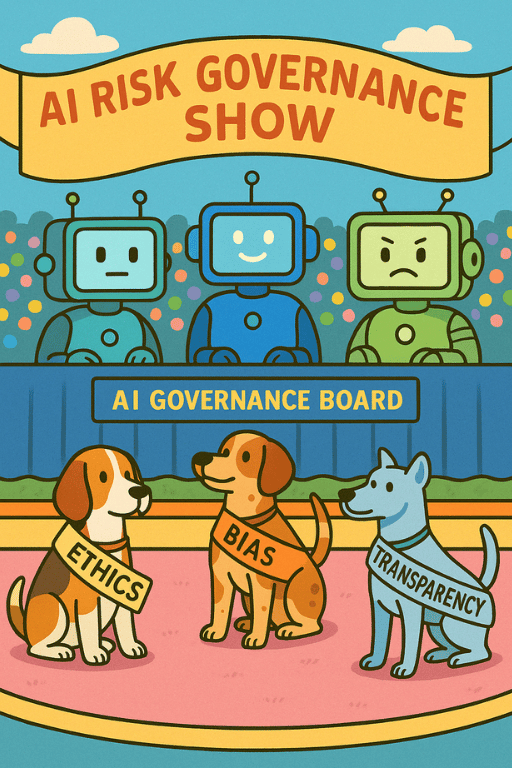
Shadow AI
Shadow AI refers to the unauthorized or unmanaged use of artificial intelligence systems within an organization, typically deployed without proper oversight, security controls, or adherence to regulatory standards. Much like “shadow IT,” these AI systems operate outside established governance structures, increasing exposure to security vulnerabilities, regulatory non-compliance, ethical issues, and data breaches.
The inclusion of comprehensive guidelines within our AI Acceptable Use Policy (AUP) to explicitly govern and mitigate Shadow AI is essential. By clearly defining permissible AI activities, mandating accountability structures, and establishing rigorous monitoring protocols, our AUP ensures that AI use remains transparent, secure, compliant, and ethically sound, protecting the organization from unintended consequences and maintaining stakeholder trust. Mitigated these by:
- Conducting Gap Analysis: Performing a gap analysis involves assessing the current state of AI usage against a defined framework for secure and governed AI implementation. This helps identify discrepancies between authorized and actual AI systems and highlights areas needing attention.The analysis should focus on trustworthiness, safety, accountability, and fairness in AI system usage and governance
- Implementing Unauthorized System Detection Mechanisms: Organizations should put in place mechanisms to actively identify AI systems that have not been formally approved or documented3 …. This can involve:
- Continuous Monitoring: Employ real-time tracking and alerting systems to detect unauthorized AI deployments.
- AI Inventory Management: Creating a Comprehensive AI System Inventory: Developing a detailed list of all AI systems in use within the organization is a fundamental step towards gaining visibility and preventing shadow AI. This inventory helps ensure that all AI systems are accounted for and can be managed appropriately. Integrating with existing asset management systems can aid in this process. The completeness of this inventory is an evaluation criterion for shadow AI prevention efforts.
- Access Control and Governance: Enforce strict access controls and periodic audits of user permissions to minimize shadow AI occurrences
Vendor and Third-Party Management
Third-party AI solutions pose significant risks if inadequately managed. Establish rigorous selection, assessment, and periodic review processes for vendors. Regularly audit vendor AI systems and document incident response procedures for third-party-related security or ethical breaches.
- Structured Audit Methodologies: Integrate regular audits using established frameworks like GAO’s AI Accountability Framework, CSA guidelines, and ISO 42001.
- Detailed Compliance Checks: Clearly define compliance requirements in contracts with third-party vendors and regularly verify adherence.
- Incident Response Enhancements: Develop robust incident detection, reporting, and remediation protocols, ensuring compliance-related issues are rapidly addressed and documented.
Training and Awareness Programs
- Develop comprehensive training programs to educate employees on the responsible use of AI.
- Conduct regular interactive sessions emphasizing practical case studies and real-world scenarios.
- Keep training sessions concise, frequent, and engaging to maximize participation and retention.
- Mandatory Responsible e‑learning (on hire + annually) covering acceptable prompts, shadow‑AI rules, data‑leak risks, bias basics and incident‑reporting lines. (Ensures every user understands their obligations in the AUP)
- Secure Sandbox for Generative‑AI Experimentation—no external streaming, limits on data‑paste size, auto‑deletion after 30 days. (Allows innovation while containing leakage and misuse.)
- “AI‑Bug‑Bounty” & Incident Hotline so employees and external researchers can report model failures or ethical concerns without retaliation. (Creates a feedback loop and supports continuous policy refinement.)
Implement ongoing communication campaigns to reinforce AI policy compliance and awareness.
Enforcement and Incident Response Procedures
Clearly define enforcement mechanisms and incident response processes:
- Document clear response workflows for policy violations and breaches.
- Establish anonymous reporting channels for policy violations and suspicious AI activity.
- Regularly communicate enforcement actions to employees to reinforce adherence and accountability.
Review and Maintenance Schedules
Regular policy reviews and updates are essential due to rapidly changing technologies and regulations:
- Conduct annual comprehensive reviews and updates.
- Perform periodic audits and assessments to identify and address gaps promptly.
- Document changes meticulously and communicate them clearly across the organization.
- Performance Metrics: Establish clear KPIs such as model accuracy, incident rates, response times, compliance adherence, and bias reduction.
- Evaluation Frameworks: Adopt standardized evaluation frameworks from CSA, NIST, and ISO to regularly assess governance effectiveness.
- Periodic Reviews: Schedule comprehensive reviews to measure progress, document lessons learned, and continuously refine governance strategies.
Practical Steps for Creating an AI AUP
- Stakeholder Engagement: Start by engaging stakeholders, including senior executives, data scientists, compliance officers, legal teams, and end-users. Understanding their perspectives ensures buy-in and addresses their specific concerns upfront.
- Risk-Based Approach: Prioritize high-risk AI applications initially. Gradually expand coverage to lower-risk areas as the policy matures and confidence grows.
- Pilot Programs and Iteration: Launch pilot implementations, gather user feedback, and continuously refine the AUP. Foster an environment of continuous improvement and adaptability.
Real-World Example: Successful Implementation
A mid-sized healthcare organization successfully implemented an AI Acceptable Use policy specifically targeting medical diagnostics AI. They established clear governance structures, data handling protocols, and rigorous ethical reviews. When facing regulatory scrutiny, they demonstrated compliance and proactive management, earning commendations and enhanced stakeholder trust.
Wrapping it up and Call to Action
Implementing a comprehensive AI Acceptable Use Policy is crucial to managing risks, complying with regulations, and building organizational resilience. The dynamic nature of AI requires ongoing vigilance and adaptability. Proactively create or refine your AI Acceptable Use policy now to ensure your organization remains compliant, responsible, and ahead of potential AI-related challenges.