AI Lifecycle Governance – Milestone 1 of 13: Objectives & Problem Definition
So, let’s get into it. AI governance can feel daunting. Trust me, I know. As AI works its way into the vast majority of organizations in some form, the looming question grows louder: How big a beast is this thing going to be?
Huuugge.
Because AI, machine learning, agentic AI, and retrieval‑augmented generation (RAG) are embedding themselves at every layer of technology stacks, applications, systems, services, and protocols. The security, compliance, privacy, and legal consequences of using these technologies become critical.
Think of AI as…
- Code that may hide bugs, vulnerabilities, and logic flaws.
- A workforce member whose roles and activities demand authorization reviews.
- An identity that must pass authentication audits and follow least‑privilege, separation‑of‑duties, and zero‑trust best practices.
- An exposed system that needs threat and penetration testing; APIs, ports, and prompt inputs can all be attacked or injected.
- A data sponge that might ingest or leak sensitive financial, health, intellectual‑property, or personal information, doing a hacker’s job before extortion even starts.
- A risk multiplier that can trigger incidents through hallucinations, giving bad advice to engineers, developers, or legal teams (like a colleague whose well-meaning mistakes create extra cleanup for everyone else).
On top of all that, AI introduces challenges around
- Bias
- Ethics
- Transparency
- Explainability
- Accountability
- Risk
No wonder the job feels overwhelming. Many leaders believe that keeping an AI use‑case tracker is “good enough.” Given where most organizations are today, that is a decent start, but it is only a start. This is a marathon, not a sprint.
That is where Tech Jacks comes in with a refreshing swig of water to keep you moving along. We deliver AI Lifecycle Governance right to your doorstep, and at a sensible price. To tame this beast, let’s first examine the seven key stages of AI lifecycle governance:
AI Lifecycle Governance Stage | Concise, Standards-Aligned Explanation |
1 · Planning & Design | Establish the business problem, success metrics, and a preliminary risk profile before development starts. Activities include use-case validation, feasibility studies, and a high-level impact assessment that sets security, privacy, and ethical guardrails. This corresponds to the Map and cross-cutting Govern functions in the NIST AI RMF, EU AI Act Article 9 risk-management duties, and ISO 42001 clauses 4 and 6 on context and planning. |
2 · Data Collection & Processing | Gather, clean, label, and document data while embedding governance, provenance checks, and bias-mitigation controls. Data sheets, lineage logs, and initial bias assessments are typical outputs. The step fulfills EU AI Act Article 10 “Data and Data Governance” requirements, the Map → Measure data categories in NIST AI RMF, and ISO 42001 Annex A data-management expectations. |
3. Model Development & Training | Select algorithms, engineer features, train, tune, and document the model with repeatability and auditability in mind. Secure ML-Ops pipelines store code, parameters, and artifacts in a governed registry. This maps to Measure model categories in NIST AI RMF, the design and development controls in ISO 42001 clause 8, and the technical-documentation duties for high-risk AI in EU AI Act Annex IV. |
4. Testing & Validation | Evaluate the model against accuracy, robustness, fairness, privacy, and security metrics, and confirm alignment with Stage 1 objectives. Independent reviewers perform adversarial and explainability tests before a go/no-go decision is made. The work aligns with Measure and performance-evaluation requirements in NIST AI RMF and ISO 42001 clause 9 performance evaluation, and underpins EU AI Act conformity assessment for high-risk systems. |
5. Deployment & Integration | Promote the validated model into production through change control, secure pipelines, and rollback plans. Ensure APIs, user interfaces, and downstream systems are compatible and that human-in-the-loop oversight is active where required. This corresponds to the Manage 1.1 deployment determination in NIST AI RMF Playbook, the operational requirements in ISO 42001 clause 8, and EU AI Act transparency and human-oversight obligations. |
6. Operation & Monitoring | Continuously monitor performance, data drift, security posture, and emerging ethical or legal risks. Incident-response runbooks, feedback loops, and periodic retraining keep the system safe, effective, and compliant over time. Stage 6 realizes the ongoing Manage cycle in NIST AI RMF, the EU AI Act post-market monitoring and incident-reporting framework (Articles 61–62), and the continual-improvement requirements in ISO 42001 clause 9 and clause 10. |
7. Retirement & Decommissioning | When the system no longer meets business, technical, or compliance needs, execute a structured wind-down: archive or delete data, migrate users, and document lessons learned. Proper decommissioning limits technical debt and legal exposure. The step is mandated by the Govern/Manage decommissioning outcomes in NIST AI RMF, lifecycle closure expectations in ISO 42001, and supports the EU AI Act’s emphasis on ongoing risk management beyond active use. |
Each stage will have a host of activities, workflows, potential forms, deliverables and tollgates. My Job will be to help break these down, and on top of providing comprehensive coverage, help create a lean (critical path) version that allows organizations to move confidentially and grow into the maturity. This allows organizations to adopt as needed, based on business requirements, available resources, and their stomach for eating the whole elephant.
Each section will have a host of activities, workflows, potential forms, deliverables and toll gates. My job will be to help break these down and on top of providing comprehensive coverage, help create a lean (critical path) version that allows organizations to move confidentially and grow into the maturity as needed based on business requirements, resources, and stomach for eating the whole elephant.
AI Lifecycle Governance Stage 1 – Planning & Design
At a high-level, we can break down Stage 1 into it’s core constituent activities listed below:
Objectives and Problem Definition
- Clearly articulate the problem the AI system aims to solve.
- Define explicit goals and objectives that are clear, specific, measurable, aligned with business opportunities, and validated through stakeholder input.
High-Level Requirements and Architecture
- Establish comprehensive high-level requirements (functional, non-functional, technical, and socio-technical), considering trustworthy AI characteristics (validity, safety, fairness, transparency, robustness, explainability).
- Outline the overall design approach and high-level architecture of the AI solution, integrating key security and privacy measures (“Security-by-Design,” “Privacy-by-Design”).
Feasibility and Use Case Validation
- Conduct initial feasibility studies to determine viability from technical, operational, ethical, regulatory, and business perspectives.
- Validate the business case, ensuring clear alignment with organizational strategies, and evaluate the expected return on investment (ROI).
Comprehensive Risk and Impact Assessment
- Conduct preliminary risk and impact assessments, incorporating a thorough identification and analysis of potential risks, including data bias, privacy violations, fairness concerns, ethical dilemmas, and security vulnerabilities.
- Categorize and rank risks according to their severity, likelihood, and impact on individuals, organizations, society, and the environment, aligning explicitly with the risk-based classifications outlined in the EU AI Act, NIST AI RMF, and ISO 42001.
Regulatory and Compliance Alignment
- Define the regulatory landscape comprehensively, explicitly addressing data privacy (GDPR, CCPA/CPRA), intellectual property considerations, industry-specific regulatory requirements, and emerging regulations like the EU AI Act.
- Map the AI system design and operational planning explicitly against relevant regulatory obligations.
Ethical and Responsible AI Framework
- Establish clear ethical principles and responsible AI guidelines based on widely recognized frameworks (OECD AI Principles, IEEE Ethically Aligned Design, ISO/IEC 23894).
- Integrate explicit ethical reviews early in the planning stage, ensuring ethical principles guide design and operational choices throughout the AI lifecycle.
Roles, Responsibilities, and Governance
- Clearly define and document roles and responsibilities for AI governance, lifecycle management, risk assessment, compliance oversight, and operational implementation.
- Identify and engage relevant AI stakeholders (internal and external) for participation in risk identification, impact assessments, and decision-making processes.
Resource and Capability Planning
- Identify and plan for necessary resources including datasets, computational infrastructure, software tooling, and human expertise for subsequent stages.
- Assess organizational capabilities and training requirements to ensure readiness for responsible AI implementation.
Data Management Protocols
- Define robust data management protocols from inception, clearly identifying data sources, privacy requirements, ethics considerations, and strategies for mitigating biases and ensuring data quality, representativeness, and provenance.
Security and Privacy Integration (by Design)
- Initiate threat modeling to proactively identify potential cybersecurity threats.
- Integrate comprehensive privacy measures early in design to address data protection and regulatory requirements effectively.
Organizational Risk Tolerance
- Clearly articulate organizational risk tolerance for AI initiatives, establishing boundaries and guidelines for acceptable risk in AI deployment and operations, and ensuring alignment with organizational risk appetite and governance structures.
Stakeholder Engagement and Transparency
- Formalize stakeholder analysis and engagement processes, documenting potential impacts on affected stakeholders (individual, organizational, societal).
- Establish transparent mechanisms for stakeholder feedback, promoting openness and accountability in alignment with NIST transparency recommendations.
Responsible Design & Development Processes
- Define specific and structured processes for responsible AI design and development aligned with best practices, including iterative evaluation and validation, accountability mechanisms, and documentation requirements.
There are 13(!) categorized activities that may spawn (and most certainly will spawn) child-tasks or deliverables that help meet the spirit of the main objectives listed.
It is recommended that you leverage as much automation and templating as possible when building your program. Models will almost always have unique elements, as will their use cases, but eventually a critical mass will be reached—at which point you can likely leverage previous work to “shortcut” evaluations that you have already deemed acceptable or low-risk based on earlier due diligence.
Now, this doesn’t mean you are not validating the risk associated with AI models and use-cases. It simply means there’s no need to perform a full, comprehensive evaluation for every request to use ChatGPT or Claude AI, especially when it’s the sixth request in a one-month period and the use-cases have 95% overlap. We work smarter, not harder.
Objectives and Problem Definition
A critical first step in the development of any AI system is to clearly articulate the problem it aims to solve. This involves identifying the specific challenges or inefficiencies that the system seeks to address, ensuring that they are well-understood and based on reliable data and real-world needs. Without a clear understanding of the problem, the risk of designing a solution that misses its mark or fails to deliver value significantly increases.
To complement a well-defined problem, explicit goals and objectives must be established. These goals should be clear, specific, and measurable, providing a concrete framework to gauge progress and success. Additionally, objectives should align closely with relevant business opportunities, fostering solutions that not only address the problem but also deliver meaningful impact. Stakeholder input plays a vital role in this process, as it ensures that the solution reflects the needs of those it serves. By validating objectives through collaborative feedback, organizations can enhance alignment, build trust, and mitigate potential missteps early in development.
Made with Visme Infographic Maker
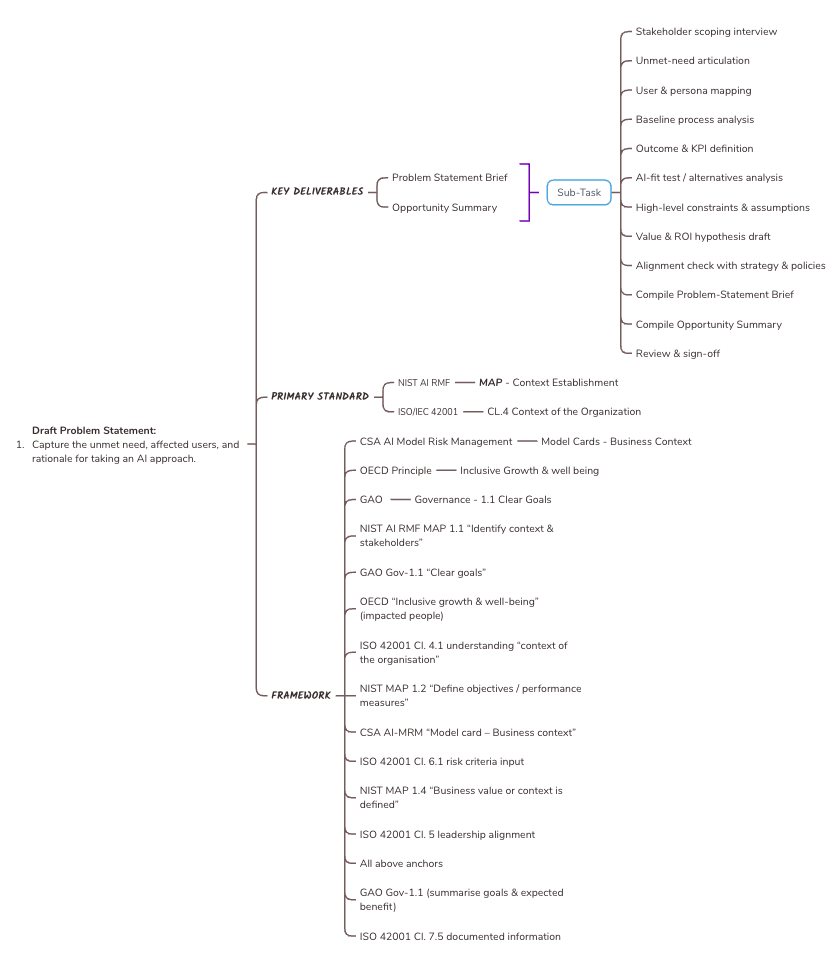
When starting an AI project, it’s tempting to dive into exciting ideas, but taking the time to draft a clear Problem Statement can completely change the trajectory of your work. It’s not just about filling out a template; it’s about creating a foundation of governance that guides every decision, milestone, and outcome across the AI Lifecycle. Think of it as setting the course before a long journey; it ensures you stay on track throughout the project.
It starts with a stakeholder-scoping interview, bringing together key voices: business sponsors, domain experts, compliance officers, and, most importantly, end-users. This step creates a community of accountability, reflecting principles from frameworks like the NIST AI RMF and the EU AI Act. These voices help shape a one-sentence unmet-need statement, a user-persona map, and a baseline process analysis. Instead of relying on assumptions, you turn intuition into evidence and anchor decisions to data like cost, cycle time, and error rates.
How often do projects derail because expectations weren’t grounded in reality? By drafting clear outcomes, testing whether AI is even the right solution, and documenting constraints, you practice “risk-based thinking” as outlined in ISO 42001. This reduces mid-project pivots and ensures defensible, well-reasoned decisions that strengthen governance across the AI Lifecycle.
By quantifying value, hypothesizing ROI, and aligning the idea with corporate strategy, privacy policies, and ethical guidelines, you integrate the technical concept into the organization’s mission. This ensures your initiative isn’t just a cool idea but a risk-aware, strategically aligned project respected by regulators and auditors. It provides traceability and demonstrates diligence throughout the AI Lifecycle.
Finally, all these insights come together in a Problem-Statement Brief and an executive-level Opportunity Summary. Routing this package through governance committees creates an official record in your GRC repository. This document serves as a roadmap and reference point for every stage of the AI Lifecycle: design, data collection, model validation, and beyond.
These steps turn an abstract idea into a measurable, business-aligned initiative. When every dataset, model checkpoint, and deployment decision ties back to a clear, approved definition of success, you’re not just building AI, you’re building trust and accountability across the entire AI Lifecycle. Isn’t that what every great project needs?
Identify Business Drivers & Value
Identifying Business Drivers and Value is like building a sturdy bridge between big, ambitious ideas and a grounded, financially sound AI initiative. It is the step where we anchor all the excitement of AI to something tangible that truly matters to the business and its goals, setting a firm foundation for the AI Lifecycle ahead.
It starts with a conversation — a stakeholder-kickoff interview that gathers every key player, at least metaphorically, and makes sure their voices are heard. Executive sponsors, finance partners, domain experts, compliance leads, and end-users all feed into a living Stakeholder Register, so no critical perspective is missed when funding decisions or governance checkpoints arise across the AI Lifecycle.
From there, we distill the noise into one simple sentence: a Need Statement. This keeps the team grounded and blocks scope creep. Then comes the baseline Metrics Sheet that captures cost, cycle time, error rates, and regulatory friction, just like taking a “before” snapshot at the start of a fitness journey.
Imagine reaching the halfway point of a project only to discover the required data does not exist or cannot be used legally. The Data Feasibility Note prevents that by confirming early that the raw material for machine learning is available and lawful. With that reality check in place, we draft an ROI hypothesis that links real numbers such as revenue growth, cost savings, or risk reduction to the vision, converting enthusiasm into a clear go-or-no-go decision. An Alternatives Analysis Memo then demonstrates why AI is the best choice compared with rules engines, RPA, or manual processes.
Transparency remains essential. A Strategy-Alignment Note verifies alignment with corporate strategy, AI ethics policies, and OECD principles, embedding trust for the entire AI Lifecycle. All insights converge into an executive Opportunity Summary and a two-page Problem-Statement Brief that guide steering-committee deliberations. Once approved, an Approval Record is archived in the GRC repository, creating a traceable audit trail.
The result is a validated business case, clear success metrics, and documented accountability that set the stage for subsequent phases of the AI Lifecycle. Building impactful AI is not just about technology; it is about delivering solutions that make a real difference, and it all starts here.
Define SMART Objectives
Defining SMART Objectives turns a big idea into a clear, actionable plan that everyone can follow. It anchors AI governance and model-risk management to measurable commitments and forms the foundation of the AI Lifecycle by setting precise goals for every phase. The process starts by analyzing the Problem-Statement Brief to extract the value the project should deliver and the pain points it must address. Each insight becomes a candidate goal, which is rewritten so it is Specific (crystal-clear action), Measurable (numeric KPI), Achievable (validated by the tech lead), Relevant (aligned with the value hypothesis), and Time-bound (deadline such as the end of Q4). Once finalized, every objective is stored in a SMART Objective Register that acts as the team’s roadmap.
For each objective the team defines a quantitative KPI, selects the proper unit of measurement, identifies a reliable data source, and records the baseline. Realistic yet ambitious targets are then set—for example, reducing false-negative rates to five percent by the end of Q4—after review with the tech lead and UX partner to balance stretch against feasibility.
A dedicated column links each KPI to Trustworthy-AI attributes such as fairness or robustness, keeping ethics in view throughout the AI Lifecycle. Dependencies and assumptions, like data quality or infrastructure scalability, are documented so blockers are visible early. When the SMART Objective and KPI table is complete, it is circulated for stakeholder comment; the steering committee signs the Objective Register, and the digitally signed file is archived to create an immutable governance record.
These KPIs are then embedded in the requirements-management system and connected to the risk register, weaving them into sprint reviews, governance dashboards, and post-deployment monitoring across the AI Lifecycle. By converting ambitions into numbers that can be tracked, the team gains a north star that guides design, development, validation, and ongoing improvement.
Vague goal | SMART objective |
---|---|
Improve model accuracy | Increase precision from 0.78 to 0.88 on the production dataset by November 30 2025 |
SMART Objectives are revisited during data-quality checks, model-performance reviews, and post-deployment audits, tying every milestone of the AI Lifecycle back to clear evidence of business value.
Set Context of Use & Boundaries
The Context of Use and Boundaries section builds a shared understanding of an AI system’s environment, users, and legal or technical constraints. This milestone completes the problem-definition phase in the Planning Stage, locking in scope so that all later steps stay aligned with agreed parameters.
It begins with a Scenario Overview, a narrative that answers three questions: Where will the system run? Who will use it—internal teams, customers, or both? What business outcome does it support? Establishing this story from the outset prevents scope creep and provides a steady reference point for all future requests.
The Decision-Stakes Table comes next, ranking each AI-influenced decision by risk. Low-impact choices receive lighter scrutiny, while high-stakes calls affecting safety, finances, or individual rights receive deeper assurance and testing.
A Persona Matrix maps user types and access channels such as web apps, edge devices, or APIs. UX designers and security engineers rely on this to align authentication flows, logging, and audit trails with real-world usage.
A High-Level Data-Flow Diagram then outlines inputs, outputs, storage locations, and external services. This single view seeds privacy reviews, threat modeling, and compliance mapping as the system evolves.
Environmental limits go into a Constraints Sheet that records latency ceilings, offline-mode requirements, and hardware caps. By grounding engineers in these realities, the sheet prevents design assumptions that fail in the field.
Legal obligations appear in a Regulatory Scope Map that spells out sector rules, geographic boundaries, and export controls. A Boundary Matrix lists explicit exclusions and non-goals to keep the project from drifting into unapproved features.
The Assumption Register logs factors like data freshness, upstream API reliability, and staffing needs, feeding directly into the broader risk-management process. Security, privacy, ethics, and UX leads review all artifacts, with findings captured in Review Minutes.
Finally, every element is consolidated into a Context-of-Use Dossier. After governance-committee sign-off, the dossier is archived as a Signed Context Approval Record, memorializing the agreed scope before design begins.
By defining where the system will operate, identifying decision stakes, and outlining legal obligations, this milestone becomes the north star for designers, developers, and auditors. It aligns every phase of the AI Lifecycle and helps ensure the solution meets business goals while satisfying regulatory requirements.
The remaining sections below will be added this week:
Preliminary Risk & Impact Scoping
Stakeholder Engagement & Requirement Elicitation
Validate Business Case & Objectives
Document & Secure Approval
This article is in progress and will receive regular updates and enhancements. It has been published unfinished in order to expedite value and visibility for the establishment of AI Lifecycle Governance practices for the community