Unleashing AI Potential Through Better Governance
Ready to unlock the power of AI without derailing into regulatory nightmares or PR disasters? You’ve come to the right place. In this guide, we’ll show you how to build an AI Governance Committee in just eight definitive steps so you can innovate, grow, and stay on the right side of compliance.
Picture a friendly roundtable of legal, compliance, ethics, security, data science, and business pros, all working together to keep your AI initiatives on track. Think of them like your internal GPS, helping you dodge bias and privacy landmines while accelerating toward real business value. Major standards like NIST, GAO, and CSA aren’t just theoretical. They’re basically cheering you on to formalize AI oversight.
A well-structured Compliance Committee and a clearly defined AI lifecycle actually supercharge value creation. By laying down guardrails early, especially around model training, deployment, and monitoring, you reduce friction, minimize rework, and free your teams to experiment confidently.
Instead of feeling like a roadblock, thoughtful governance becomes something else entirely: the premium octane that lets everyone move faster.
AI’s potential as a competitive game-changer is off the charts, but racing ahead blindly is a lot like speeding in a school zone: you might outpace your rivals for a moment, but you also run a higher risk of getting nabbed by regulators. In short, an AI Governance Committee is how you innovate rapidly and responsibly: building trust and minimizing chaos along the way.
Kicking off by Establishing Objectives
1. Establish the Mandate and Objectives:
The initial and paramount step is to clearly define the purpose, scope, and objectives of the AI Governance Committee. This involves understanding why the committee is being formed and what it is expected to achieve in relation to the organization’s AI initiatives. This stage should also involve obtaining clear support and a mandate from senior leadership and the board of directors, ensuring the committee has the necessary authority to be effective. Defining clear goals and objectives for AI systems themselves will also inform the committee’s priorities. This aligns directly to NIST AI RMF GOVERN principal
2. Define the Committee’s Composition and Roles:
The next critical step is to identify and appoint members to the AI Governance Committee, ensuring a multidisciplinary representation from relevant departments across the organization. This committee should include technical teams (AI Development, IT Security, Data Science), business units, legal and compliance, risk management, and potentially ethics experts and executive leadership Simultaneously, clearly define the roles, responsibilities, and reporting structure of the committee and its members, potentially utilizing a RACI model to ensure accountability and clarity. This includes determining who is responsible for what aspects of AI governance, who is accountable for decisions, who needs to be consulted, and who needs to be informed.
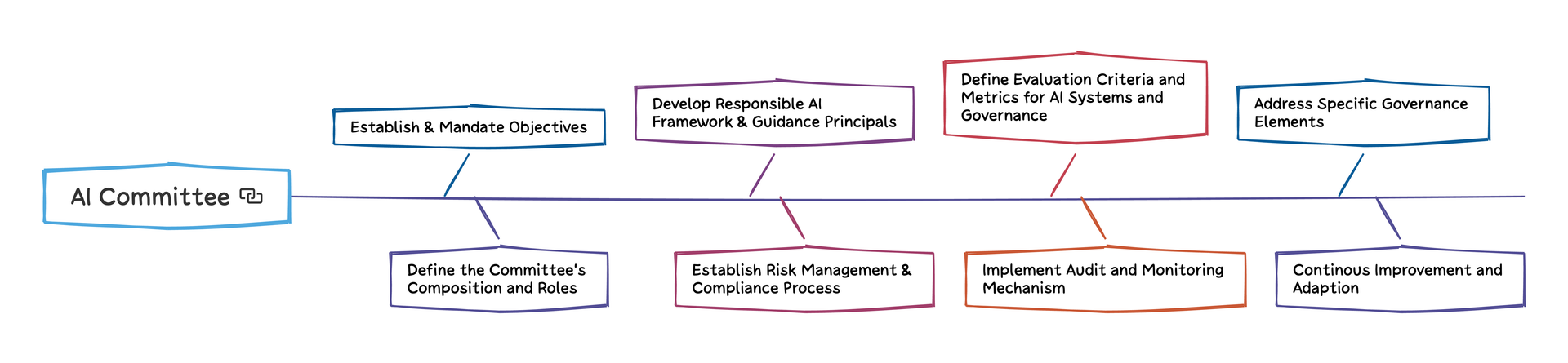
At a High-Level, the 8-steps above serve as a guiding outline for the core functions that the AI Committee will oversee. Your organization may not need every function. In fact, your organization may need to create its own unique and business specific functions, so feel free to adjust as needed. This is where an AI Governance Charter can come in helpful, as it can be used to streamline your organizations need based on your own intimate knowledge of business requirements.
Maturing the Committee’s Function
As the Committee becomes more established and the organization’s AI Inventory, Use Cases, and compliance requirements are defined, the need to assess risk increases. Now is the time to ensure that your practices align with both ethical standards and measurable impact.
3. Develop a Responsible AI Framework and Guiding Principles:
A high priority is the creation and approval of a comprehensive Responsible AI framework. This framework should outline the organization’s ethical principles, values, and guidelines for the development and deployment of AI technologies. This should address key areas such as fairness, bias mitigation, transparency, accountability, security, privacy, and societal impact. The AI Governance Committee will likely play a central role in developing and maintaining this framework. This may draw upon existing frameworks and principles like the OECD AI Principles, NIST AI RMF, ISO/IEC 42001 etc.
By referencing widely adopted frameworks, you ensure your policies resonate across diverse teams and keep your AI aligned with global standards.
Without a cohesive framework, your teams might adopt conflicting policies on data privacy or bias mitigation, leading to stalled projects or even public missteps that tarnish your brand
4. Establish Risk Management and Compliance Processes:
Implementing robust risk assessment and risk management methodologies specific to AI is crucial. This step involves identifying, analyzing, and prioritizing AI-related risks across the AI lifecycle. The committee should also establish processes to ensure compliance with relevant laws, regulations, and industry standards. This includes defining security objectives and measurable controls.
If you can’t articulate potential risks upfront (for example, how an AI model might inadvertently discriminate in a lending application), you could face lawsuits or fines after the damage is done.
5. Define Evaluation Criteria and Metrics for AI Systems and Governance
To ensure accountability and effectiveness, the committee needs to establish quantifiable evaluation criteria and key performance indicators (KPIs) for both the AI systems being developed/integrated and the AI governance processes themselves. These metrics will help in assessing the performance, reliability, security, ethical compliance, and overall impact of AI initiatives, as well as the effectiveness of the governance framework.
Tracking metrics like model accuracy, false positives/negatives, or fairness scores can detect performance dips early, preventing costly rollbacks or reputational damage.
Similarly, monitor the governance process itself by measuring how long it takes to review compliance for new AI projects. This keeps your team from getting bogged down in red tape and helps maintain a balance between rapid innovation and proper oversight.
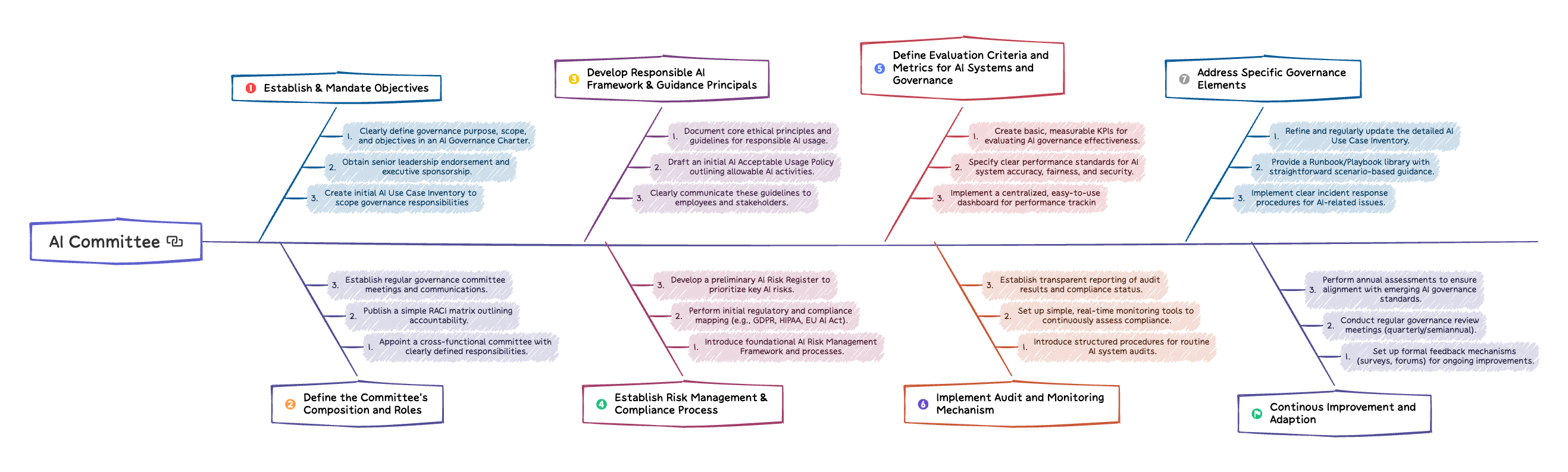
Full Governance Engaged
With a solid framework and defined metrics in place, it’s time to operationalize full-scale governance that covers auditing, specialized controls, and continuous improvement.
6. Implement Audit and Monitoring Mechanisms:
Establishing processes for regular audits of AI systems and governance practices is essential for ongoing oversight and compliance. This includes defining the audit scope, assigning audit ownership, developing audit methodologies, and setting audit metrics.
Furthermore, implementing continuous monitoring and reporting mechanisms for AI system performance, risks, and adherence to policies is vital for early detection of issues and proactive management.
Without scheduled audits and transparent logging, unapproved changes or security gaps can remain hidden, making it harder to detect and address issues before they escalate. Consistent monitoring builds trust with stakeholders—when regulators or senior leadership see robust evidence of oversight, they’re more likely to support new AI initiatives.
7. Address Specific Governance Elements:
Once the foundational elements are in place, the committee can prioritize specific governance elements such as:
Shadow AI Prevention: Implementing measures to identify and govern unauthorized AI systems within the organization.
Data Governance: Focusing on data quality, security, privacy, and ethical use in AI systems.
Model Governance: Establishing policies and procedures for the development, deployment, monitoring, and retirement of AI models. This includes aspects like version control, performance monitoring, and addressing model drift.
Access Control: Implementing mechanisms to manage and restrict access to AI systems, models, and data based on roles and responsibilities.
Output Evaluation and Guardrails: Establishing mechanisms to assess and control the outputs of AI systems to ensure safety, accuracy, and alignment with organizational values.
Third-Party/Supply Chain Management: Developing processes for assessing and managing risks associated with AI vendors and third-party components. This includes vendor assessments, contract management, and dependency monitoring.
Employee Use of GenAI Tools: Establishing policies and guidelines for the responsible and secure use of generative AI tools by employees.
Incident Response: Developing specific plans and procedures for addressing AI-related incidents.
Change Management: Implementing formal processes for managing changes to AI systems
Transparency and Explainability: Ensuring appropriate levels of transparency in AI capabilities and limitations, and striving for explainability of AI outputs where necessary
If shadow AI systems or untracked data sources crop up, your organization risks compliance breaches, ethical lapses, and wasted investment in duplicate or unauthorized projects. Clear guardrails around data usage, model oversight, and third-party risks help maintain consistent standards across teams. They ensure no one goes rogue and jeopardizes the entire AI program.
8. Continuous Improvement and Adaptation:
AI technology and its associated risks are constantly evolving. Therefore, the AI Governance Committee must prioritize continuous monitoring, review, and updating of the AI governance framework, policies, and procedures. This includes staying informed about emerging AI regulations, best practices, and new security threats. Establishing feedback loops with relevant stakeholders is also crucial for ongoing improvement.
Rapid AI advancements mean new vulnerabilities can emerge overnight. If you’re not iterating on policies and best practices, you’ll always be playing catch-up.
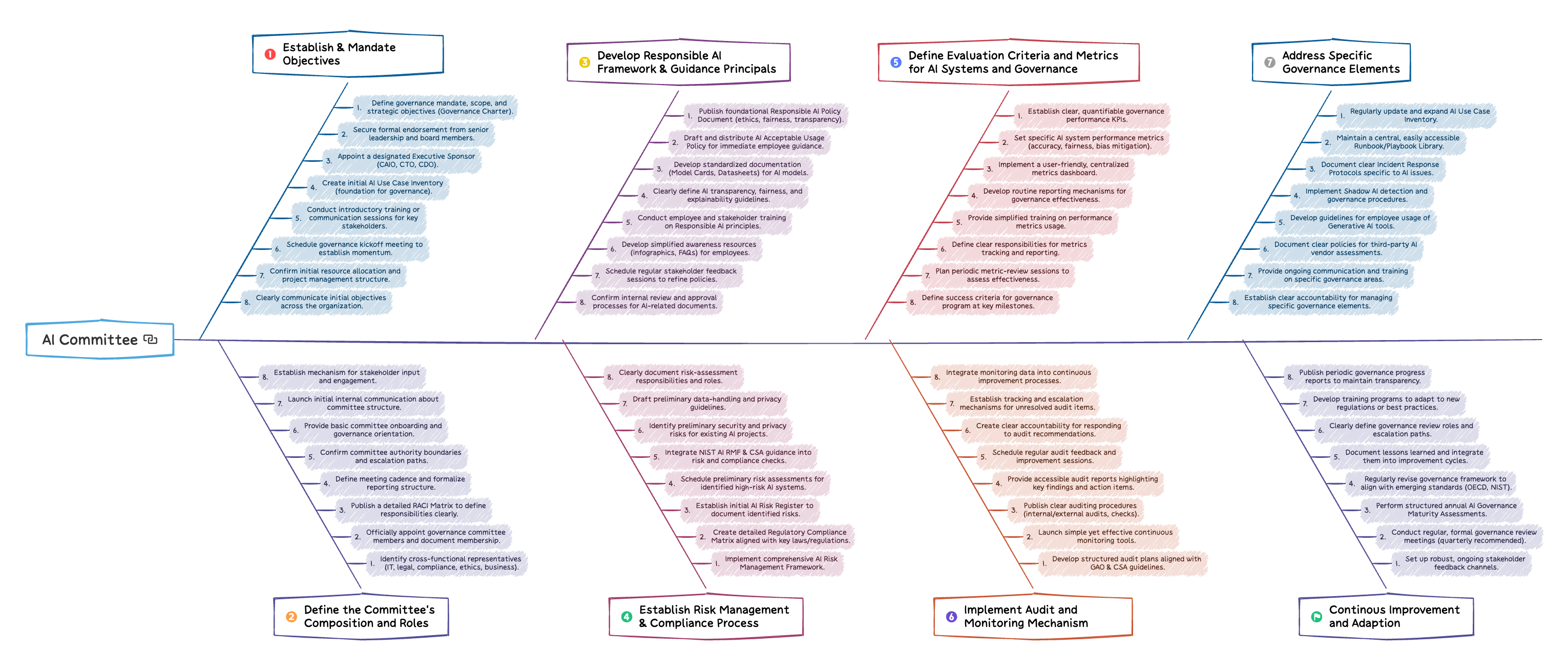
Wrapping Up
Developing vs. Consuming AI
While these governance steps apply broadly, keep in mind that organizations creating their own large language models (LLMs) may need deeper controls around data pipelines, model security, and specialized testing for bias. On the other hand, businesses consuming AI services or off-the-shelf models will focus more on vendor due diligence, contractual compliance, and ensuring data fed into these third-party tools meets organizational standards
With these measures, your AI Governance Committee isn’t just reacting to challenges; it’s actively shaping the responsible, ethical, and forward-thinking AI landscape your organization needs.
Please share and/or contact us for consultation to help educate, train, and support your business.